File System Access (FSA) API enables web applications to interact with files on the users’ local devices. Even though it can be used to develop rich web applications, it greatly extends the attack surface, which can be abused by adversaries to cause significant harm. In this paper, for the first time in the literature, we extensively study this new attack vector that can be used to develop a powerful new ransomware strain over a browser. Using the FSA API and WebAssembly technology, we demonstrate this novel browser-based ransomware called RØB as a malicious web application that encrypts the user’s files from the browser. We use RØB to perform impact analysis with different OSs, local directories, and antivirus solutions as well as to develop mitigation techniques against it. Our evaluations show that RØB can encrypt the victim’s local files including cloud-integrated directories, external storage devices, and network-shared folders regardless of the access limitations imposed by the API. Moreover, we evaluate and show how the existing defense solutions fall short against RØB in terms of their feasibility. We propose three potential defense solutions to mitigate this new attack vector. These solutions operate at different levels (i.e., browser-level, file-system-level, and user-level) and are orthogonal to each other. Our work strives to raise awareness of the dangers of RØB-like browser-based ransomware strains and shows that the emerging API documentation (i.e., the popular FSA) can be equivocal in terms of reflecting the extent of the threat.
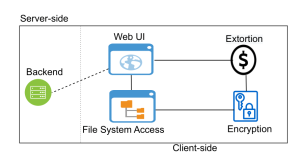
CSPoweR-Watch: A Cyber-Resilient Residential Power Management System
Modern Energy Management Systems (EMS) are becoming increasingly complex in order to address the urgent issue of global energy consumption. These systems retrieve vital information from various Internet-connected devices in a smart grid to function effectively. However, due to the critical tasks they perform and the sensitive information they handle, such devices make a very attractive target for attackers. Numerous factors including high interconnectivity and outdated firmware, result in such devices being susceptible to cyber attacks. Malicious actors can exploit these vulnerabilities present in the devices to perform detrimental tasks such as modifying critical firmware, sending bogus sensor data, or stealing sensitive information. To address this issue, we propose a novel framework that integrates PowerWatch, a solution that detects compromised devices in the smart grid, with Cyber-secure Power Router (CSPR), a modern energy management system that is connected to various smart-grid devices. In addition, we propose a distributed infrastructure scheme in which numerous CSPR devices are being monitored using PowerWatch capabilities. The goal is to ascertain whether or not CSPR has operated maliciously, which PowerWatch achieves by utilizing a machine learning model that analyzes information from system or library call lists extracted from CSPR in order to detect malicious activity in the EMS. To test the efficacy of our framework, a number of unique attack scenarios were performed on a realistic testbed that comprises functional versions of CSPR and PowerWatch. Our performance evaluation investigates the effectiveness of this first-of-its-kind merger and provides insight into the feasibility of developing future cyber-secure EMS. The results of our experimental procedures yielded 100\% accuracy for each of the attack scenarios. Finally, our implementation demonstrates that the integration of PowerWatch and CSPR is effective and yields minimal overhead to the EMS.
Project Sponsor: National Science Foundation
The High Definition Multimedia Interface (HDMI) is the backbone and the de-facto standard for Audio/Video interfacing between video-enabled devices. Today, almost tens of billions of HDMI devices exist in the world and are widely used to distribute A/V signals in smart homes, offices, concert halls, and sporting events making HDMI one of the most highly deployed systems in the world. An important component in HDMI is the Consumer Electronics Control (CEC) protocol, which allows for the interaction between devices within an HDMI distribution network. Nonetheless, existing network security mechanisms only protect traditional networking components, leaving HDMI-Walk outside of their scope. In this work, we identify and tap into CEC protocol vulnerabilities, using them to implement realistic proof-of-work attacks on HDMI distribution networks.
Whether it is one-factor or MFA, a one-time login process does not guarantee that the identified user is the real user throughout the login session. Even if it is an insider who has been authorized once, forever access is provided in most cases not to interrupt the current user. Hence, an authentication that re-verifies the user periodically without breaking the continuity of the session is vital. In this project, we introduce a Wearable-Assisted Continuous Authentication framework called WACA, where a wearable device (e.g., smartwatch) is used to authenticate a computer user continuously utilizing the motion sensors of the smartwatch.
Broadly defined as the Internet of Things (IoT), the growth of commodity devices that integrate physical processes with digital connectivity has had profound effects on society—smart homes, personal monitoring devices, enhanced manufacturing, and other IoT applications have changed the way we live, play and work. Yet extant IoT platforms provide few means of evaluating the use (and potential misuse) of sensitive information. In this project, we present SaINT, a tool for analyzing sensitive data leakage in IoT implementations. SaINT operates in three phases; (a) translation of platform-specific source code into an IR modeling sensor-computation-actuator structures, (b) identifying sensitive sources and sinks, and (c) performing static analysis to identify sensitive data leakage.
Proviz is an Internet of Things application development software. You can use Proviz to create Internet of Things applications without requiring any software or hardware experiences.
Modern devices have become “smart” in recent years with the advancement of modern electronics and wireless communication systems. Smart devices such as smartphone, smartwatch, fitness trackers, etc. are equipped with high precision sensors, empowering them to gather information about user characteristics as well as the surrounding environment. These sensor-enriched devices have opened a new domain of sensing-enabled applications. With the rapid growth sensing-enabled applications, smart devices are integrated into every possible application domain, from home security to health care to the military. Some applications can even learn the characteristics of users using sensor data and take automatic decisions to improve the user experience.
The core concept of the smart grid is the realization of two-way communications between smart devices. The integration of complex and heterogeneous networks as well as their devices into the smart grid must be done not only in an efficient but also in a secure manner. Nonetheless, with all its dependency upon device operations and communications, the smart grid is highly vulnerable to any security risk stemming from devices. Especially, the use of compromised devices can wreak havoc on the smart grid’s critical functionalities and can cause catastrophic consequences to the integrity of the smart grid data and/or users’ privacy.
Authors: Abbas Acar, Hossein Fereidooni, Tigist Abera, Amit Kumar Sikder, Markus Miettinen, Hidayet Aksu, Mauro Conti, Ahmad-Reza Sadeghi, A. Selcuk Uluagac Link: https://arxiv.org/pdf/1808.02741.pdf Corresponding Author: Abbas Acar In this work, we introduce a novel multi-stage privacy attack against user privacy in a smart environment. It is realized utilizing state-of-the-art machine-learning approaches for detecting and identifying particular types of IoT devices, their actions, states, and ongoing user activities in a cascading style by only observing passively the traffic from smart home devices and sensors. The attack effectively works on both encrypted and unencrypted communications. We evaluate the efficiency of the attack with real measurements from an extensive set of popular off-the-shelf smart home IoT devices and sensors utilizing a set of diverse network protocols like WiFi, ZigBee, and BLE. Our results show that an adversary passively sniffing the traffic can achieve very high accuracy (above 90%) in identifying the state and actions of targeted smart home devices and their users. To protect against this privacy leakage, we also propose a countermeasure based on generating spoofed traffic to hide the real activities of the devices. We also demonstrate that the provided solution provides better protection than existing solutions. 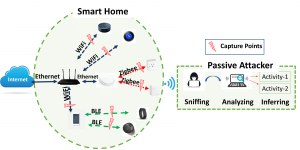
Our everyday lives are expanding fast with the introduction of new Smart Home Systems (SHSs). Today, a myriad of SHS devices and applications are widely available to users and have already started to re-define our modern lives. Smart home users utilize the apps to control and automate such devices. Users can develop their own apps or easily download and install them from vendor-specific app markets. App-based SHSs offer many tangible benefits to our lives, but also unfold diverse security risks. Several attacks have already been reported for SHSs. However, current security solutions consider smart home devices and apps individually to detect malicious actions rather than the context of the SHS as a whole. The existing mechanisms cannot capture user activities and sensor-device-user interactions in a holistic fashion. To address these issues, in this project, we introduce Aegis, a novel context-aware security framework to detect malicious behavior in a SHS.